Publications
Journal Articles
2023
Chayanika Sharma; Nairit Barkataki; Utpal Sarma
A Deep Neural Network with Electronic Nose for Water Stress Prediction in Khasi Mandarin Orange Plants Journal Article
In: Measurement Science and Technology, vol. 34, no. 12, pp. 125152, 2023, ISSN: 1361-6501.
Abstract | BibTeX | Tags: artificial intelligence, Artificial neural network (ANN), classification, water stress | Links:
@article{sharma2023deep,
title = {A Deep Neural Network with Electronic Nose for Water Stress Prediction in Khasi Mandarin Orange Plants},
author = {Chayanika Sharma and Nairit Barkataki and Utpal Sarma},
url = {https://nairit.in/wp-content/uploads/2023_MST_Sharma_Deep.pdf},
doi = {10.1088/1361-6501/acf8e3},
issn = {1361-6501},
year = {2023},
date = {2023-09-21},
urldate = {2023-09-01},
journal = {Measurement Science and Technology},
volume = {34},
number = {12},
pages = {125152},
publisher = {IOP Publishing},
abstract = {Water stress is a significant environmental factor that hampers plant productivity and leads to various physiological and biological changes in plants. These include modifications in stomatal conductance and distribution, alteration of leaf water potential & turgor loss, altered chlorophyll content, and reduced cell expansion and growth. Additionally, water stress induces changes in the emission of volatile organic compounds across different parts of the plants. This study presents the development of an electronic nose (E-nose) system integrated with a deep neural network (DNN) to detect the presence and levels of water stress induced in Khasi Mandarin Orange plants. The proposed approach offers an alternative to conventional analytical methods that demand expensive and complex laboratory facilities. The investigation employs the leaf relative water content (RWC) estimation, a conventional technique, to evaluate water stress induction in the leaves of 20 plants collected over a span of 9 days after stopping irrigation. Supervised pattern recognition algorithms are trained using the results of RWC measurement, categorising leaves into non-stressed or one of four stress levels based on their water content. The dataset used for training and optimising the DNN model consists of 27 940 samples. The performance of the DNN model is compared to traditional machine learning methods, including linear and radial basis function support vector machines, k-nearest neighbours, decision tree, and random forest. From the results, it is seen that the optimised DNN model achieves the highest accuracy of 97.59% in comparison to other methods. Furthermore, the model is validated on an unseen dataset, exhibiting an accuracy of 97.32%. The proposed model holds the potential to enhance agricultural practices by enabling the detection and classification of water stress in crops, thereby aiding in water management improvements and increased productivity.},
keywords = {artificial intelligence, Artificial neural network (ANN), classification, water stress},
pubstate = {published},
tppubtype = {article}
}

Nairit Barkataki; Banty Tiru; Utpal Sarma
Size estimation of underground targets from GPR frequency spectra: A deep learning approach Journal Article
In: Journal of Applied Geophysics, vol. 213, pp. 105009, 2023, ISSN: 0926-9851.
Abstract | BibTeX | Tags: Archeology, Artificial Neural Network, Bridge inspection, Civil Engineering, Deep learning, Ground penetrating radar, neural networks, object size prediction | Links:
@article{barkataki2023size,
title = {Size estimation of underground targets from GPR frequency spectra: A deep learning approach},
author = {Nairit Barkataki and Banty Tiru and Utpal Sarma},
url = {https://nairit.in/wp-content/uploads/Paper009_JAG_ObjectSizeAScan.pdf},
doi = {10.1016/j.jappgeo.2023.105009},
issn = {0926-9851},
year = {2023},
date = {2023-06-01},
urldate = {2023-06-01},
journal = {Journal of Applied Geophysics},
volume = {213},
pages = {105009},
abstract = {GPR (Ground Penetrating Radar) is a robust and effective device for identifying underground artefacts. Construction companies and civil engineers should be aware of the sizes of rebars and pipelines before and during construction work for various reasons. Most research efforts have typically concentrated on GPR signal analysis in the time domain, however recent studies have increasingly focused on analysis in the frequency domain. This paper proposes an artificial neural network (ANN) model for estimating the diameter of underground rods (solid) and pipes (hollow). GPR data captured in the time domain domain is transformed to the frequency domain using FFT, after which feature extraction is performed using ANN. An FPGA-based prototype GPR system is used to collect GPR A-Scan data for a variety of targets made of aluminium, stainless steel, rebar, and PVC. A mean absolute percentage error of 1.89% is achieved using the proposed model. The experimental results confirm the effectiveness of the proposed approach in extracting size-related information from GPR data.},
keywords = {Archeology, Artificial Neural Network, Bridge inspection, Civil Engineering, Deep learning, Ground penetrating radar, neural networks, object size prediction},
pubstate = {published},
tppubtype = {article}
}
2022

Nairit Barkataki; Banty Tiru; Utpal Sarma
A CNN model for predicting size of buried objects from GPR B-Scans Journal Article
In: Journal of Applied Geophysics, vol. 200, pp. 104620, 2022, ISSN: 0926-9851.
BibTeX | Tags: CNN, Deep learning, Ground penetrating radar, object size prediction | Links:
@article{barkataki2022cnn,
title = {A CNN model for predicting size of buried objects from GPR B-Scans},
author = {Nairit Barkataki and Banty Tiru and Utpal Sarma},
url = {https://nairit.in/wp-content/uploads/2023/01/Paper003_JAG_ObjectSizeDL.pdf},
doi = {10.1016/j.jappgeo.2022.104620},
issn = {0926-9851},
year = {2022},
date = {2022-01-01},
urldate = {2022-01-01},
journal = {Journal of Applied Geophysics},
volume = {200},
pages = {104620},
publisher = {Elsevier},
keywords = {CNN, Deep learning, Ground penetrating radar, object size prediction},
pubstate = {published},
tppubtype = {article}
}
2021
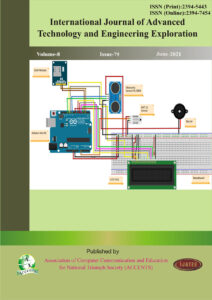
Nairit Barkataki; Banty Tiru; Utpal Sarma
Performance investigation of patch and bow-tie antennas for ground penetrating radar applications Journal Article
In: International Journal of Advanced Technology and Engineering Exploration, vol. 8, no. 79, pp. 753–765, 2021, ISSN: 2394-7454.
BibTeX | Tags: Antenna, Bow-tie, Ground penetrating radar, Patch, Slot | Links:
@article{barkataki2021performance,
title = {Performance investigation of patch and bow-tie antennas for ground penetrating radar applications},
author = {Nairit Barkataki and Banty Tiru and Utpal Sarma},
doi = {10.19101/IJATEE.2021.874072},
issn = {2394-7454},
year = {2021},
date = {2021-06-01},
urldate = {2021-06-01},
journal = {International Journal of Advanced Technology and Engineering Exploration},
volume = {8},
number = {79},
pages = {753--765},
keywords = {Antenna, Bow-tie, Ground penetrating radar, Patch, Slot},
pubstate = {published},
tppubtype = {article}
}
Conference Proceedings
2023
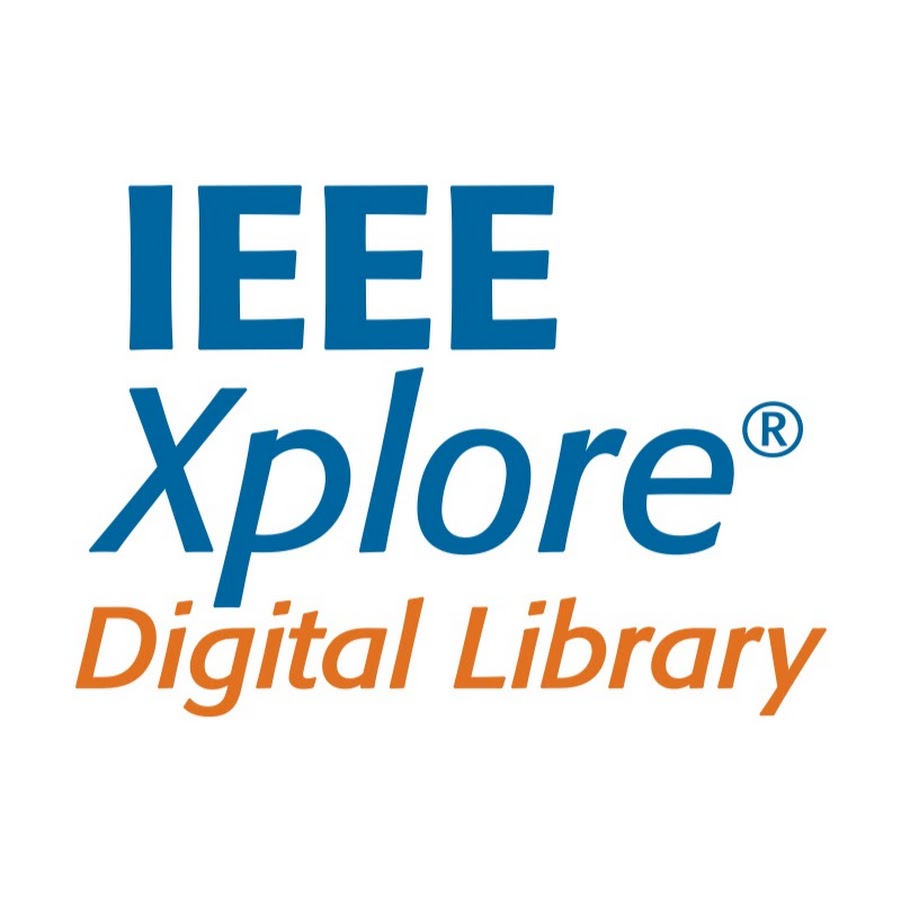
Nairit Barkataki; Pritha Mahanta; Sharmistha Mazumdar; Utpal Sarma
Efficient Hyperbola Detection and Fitting using Image Processing Techniques and Column-Connection Clustering Best Paper Proceedings Article
In: 2023 Second International Conference on Advances in Computational Intelligence and Communication (ICACIC), IEEE, 2023, ISBN: 9798350318456.
Abstract | BibTeX | Tags: Automation, C3, Column-Connection Clustering, Edge Detection Algorithm, Ground penetrating radar, Hyperbola Detection, object detection, Sub-Surface Imaging | Links:
@inproceedings{barkataki2023efficient,
title = {Efficient Hyperbola Detection and Fitting using Image Processing Techniques and Column-Connection Clustering},
author = {Nairit Barkataki and Pritha Mahanta and Sharmistha Mazumdar and Utpal Sarma},
url = {https://nairit.in/wp-content/uploads/2023_ICACIC_Barkataki_Hyperbola.pdf},
doi = {10.1109/ICACIC59454.2023.10435037},
isbn = {9798350318456},
year = {2023},
date = {2023-12-08},
urldate = {2023-12-08},
booktitle = {2023 Second International Conference on Advances in Computational Intelligence and Communication (ICACIC)},
publisher = {IEEE},
abstract = {Ground Penetrating Radar (GPR) is a preferred non-destructive method used for the identification and localisation of subsurface targets. Hyperbolic signatures present in GPR B-Scans can provide valuable insights about the shape, material and the distance at which target objects are buried below the surface. This paper presents an innovative approach to enhance GPR data analysis, specifically focusing on hyperbola detection within GPR B-Scans. Preprocessing steps, such as dewow filtering, frequency filtering, and gain compensation, are used to improve GPR data quality. A comparative analysis is conducted on the performance of Canny, Sobel, and Scharr edge detectors in identifying hyperbolic signatures. By merging the Canny edge detection algorithm with the Column-Connection Clustering (C3) method, common limitations of conventional clustering, particularly their sensitivity to noise and outliers are addressed. Finally, the efficacy of the proposed method is evaluated by calculating the R-squared values of the fitted hyperbolas which was found to be > 0.93.},
keywords = {Automation, C3, Column-Connection Clustering, Edge Detection Algorithm, Ground penetrating radar, Hyperbola Detection, object detection, Sub-Surface Imaging},
pubstate = {published},
tppubtype = {inproceedings}
}
2022
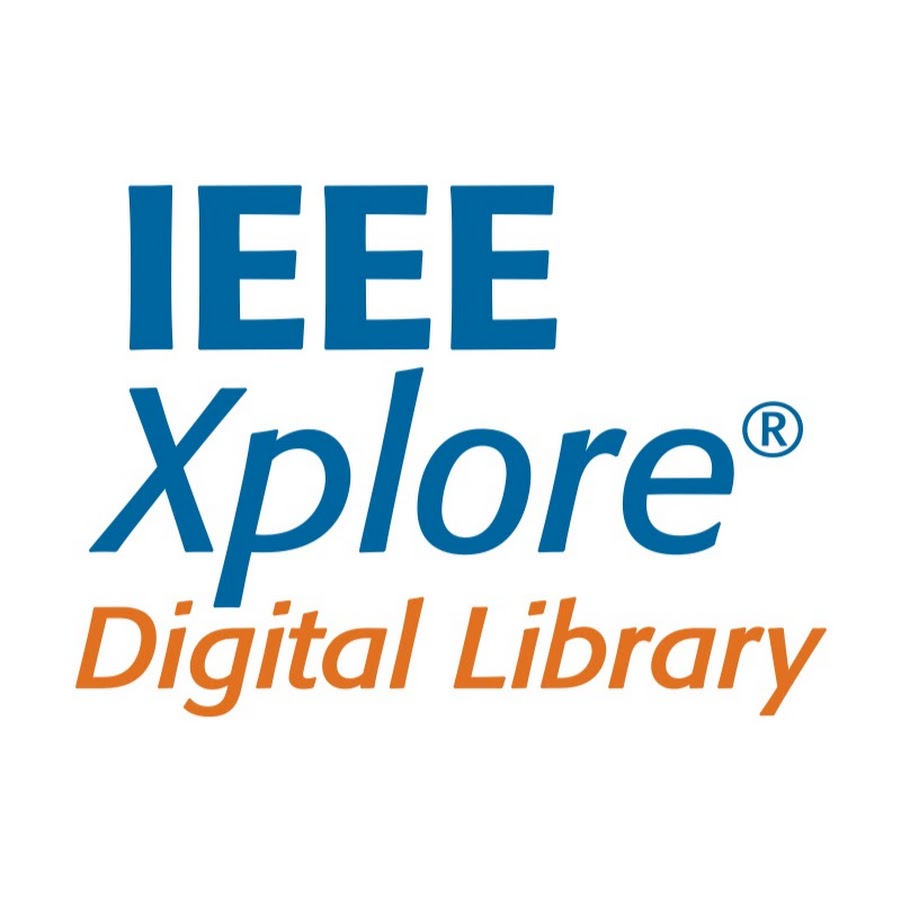
Nairit Barkataki; Ankur Jyoti Kalita; Utpal Sarma
Automatic Material Classification of Targets from GPR Data using Artificial Neural Networks Proceedings Article
In: 2022 IEEE Silchar Subsection Conference (SILCON), IEEE 2022, ISBN: 978-1-6654-7100-8.
Abstract | BibTeX | Tags: Archeology, Artificial Neural Network, classification, Deep learning, Ground penetrating radar, Landmine detection, Object Material | Links:
@inproceedings{barkataki2022automatic,
title = {Automatic Material Classification of Targets from GPR Data using Artificial Neural Networks},
author = {Nairit Barkataki and Ankur Jyoti Kalita and Utpal Sarma},
url = {https://nairit.in/wp-content/uploads/2022_SILCON_Barkataki_Material.pdf},
doi = {10.1109/SILCON55242.2022.10028944},
isbn = {978-1-6654-7100-8},
year = {2022},
date = {2022-11-06},
urldate = {2022-11-06},
booktitle = {2022 IEEE Silchar Subsection Conference (SILCON)},
journal = {2022 IEEE Silchar Subsection Conference (SILCON)},
organization = {IEEE},
abstract = {Ground penetrating radar (GPR) is a preferred non-destructive method to study and identify buried objects in the field of geology, civil engineering, archaeology, military, etc. Landmines are now largely composed of plastic and other non-metallic materials, while archaeologists must deal with buried artefacts such as ceramics, pillars, and walls built of a range of materials. As a result, understanding the material properties of buried artefacts is critical. This study presents an ANN model for automatic classification of buried objects from GPR A-Scan data. The proposed ANN model is trained and validated using a synthetic dataset generated using gprMax. The model performs well in classifying three different object classes of aluminium, iron and limestone, while achieving an overall accuracy of 95%.},
keywords = {Archeology, Artificial Neural Network, classification, Deep learning, Ground penetrating radar, Landmine detection, Object Material},
pubstate = {published},
tppubtype = {inproceedings}
}
2021
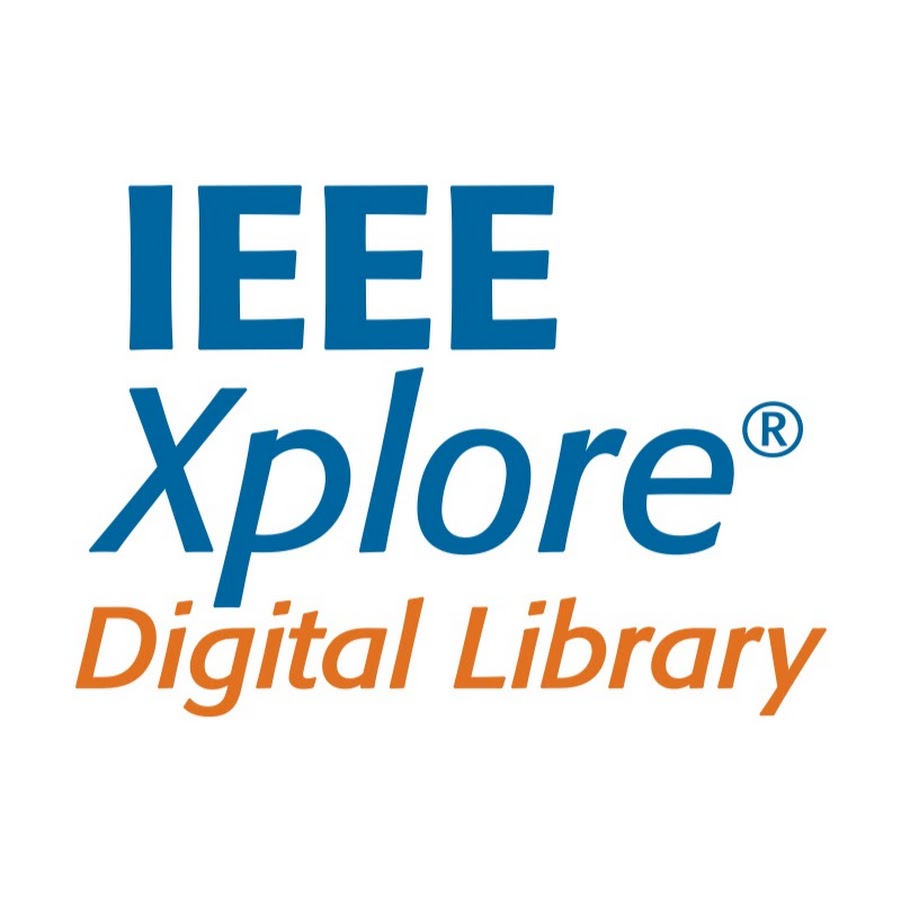
Nairit Barkataki; Sharmistha Mazumdar; Banty Tiru; Utpal Sarma
Estimation of soil moisture from GPR data using artificial neural networks Proceedings Article
In: 2021 IEEE International Conference on Technology, Research, and Innovation for Betterment of Society (TRIBES), IEEE 2021, ISBN: 978-1-6654-3342-6.
Abstract | BibTeX | Tags: Artificial Neural Network, Deep learning, Ground penetrating radar, Soil Moisture | Links:
@inproceedings{barkataki2021estimationsoil,
title = {Estimation of soil moisture from GPR data using artificial neural networks},
author = {Nairit Barkataki and Sharmistha Mazumdar and Banty Tiru and Utpal Sarma},
url = {https://nairit.in/wp-content/uploads/2022/12/149_Barkataki.pdf},
doi = {10.1109/TRIBES52498.2021.9751623},
isbn = {978-1-6654-3342-6},
year = {2021},
date = {2021-12-19},
urldate = {2021-12-19},
booktitle = {2021 IEEE International Conference on Technology, Research, and Innovation for Betterment of Society (TRIBES)},
organization = {IEEE},
abstract = {Soil moisture estimation is essential for understanding the water cycle and its impact on weather and climate. GPR based soil moisture estimation is non-invasive in nature and provides quicker results as compared to standard laboratory approaches. Deep learning algorithms have been shown to be an effective method for extracting characteristics from GPR data. To assess soil moisture, this paper offers a deep artificial neural network (ANN) model. The data set was created using finite-difference-time-domain (FDTD) simulation and is used to train and validate the ANN model. The suggested model predicts soil moisture from GPR A-Scans well, with R2 = 0.998 and mean average percentage error (MAPE) of 1.23.},
keywords = {Artificial Neural Network, Deep learning, Ground penetrating radar, Soil Moisture},
pubstate = {published},
tppubtype = {inproceedings}
}
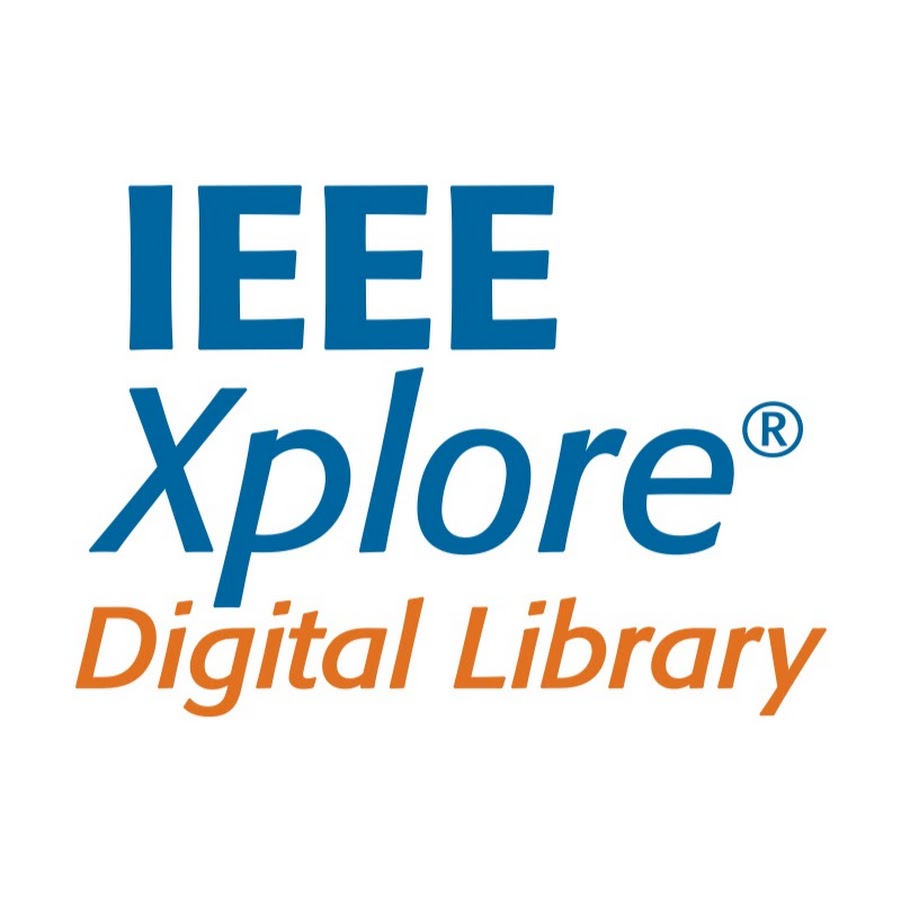
Nairit Barkataki; Pranjal Borah; Banty Tiru; Utpal Sarma
Design of a 400 MHz Cavity Backed CPW Fed Bow-Tie Antenna for GPR Applications Proceedings Article
In: 2021 International Conference on Industrial Electronics Research and Applications (ICIERA), IEEE 2021.
BibTeX | Tags: Antenna, Bow-tie, Ground penetrating radar | Links:
@inproceedings{barkataki2021design,
title = {Design of a 400 MHz Cavity Backed CPW Fed Bow-Tie Antenna for GPR Applications},
author = {Nairit Barkataki and Pranjal Borah and Banty Tiru and Utpal Sarma},
url = {https://nairit.in/wp-content/uploads/2022/12/124_NairitBarkataki_corrected.pdf},
doi = {10.1109/ICIERA53202.2021.9726755},
year = {2021},
date = {2021-12-01},
urldate = {2021-12-01},
booktitle = {2021 International Conference on Industrial Electronics Research and Applications (ICIERA)},
organization = {IEEE},
keywords = {Antenna, Bow-tie, Ground penetrating radar},
pubstate = {published},
tppubtype = {inproceedings}
}
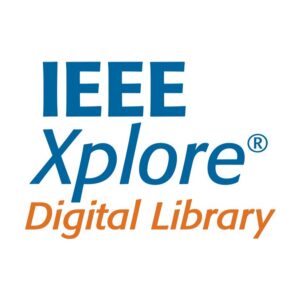
Nairit Barkataki; Anirban Bhattacharjee; Sharmistha Mazumdar; Banty Tiru; Utpal Sarma
Artificial Neural Network based automatic classification of target shapes from GPR data Best Paper Proceedings Article
In: 2021 IEEE International Conference on Computation System and Information Technology for Sustainable Solutions (CSITSS), IEEE 2021.
BibTeX | Tags: Artificial Neural Network, classification, Deep learning, Ground penetrating radar, object shape | Links:
@inproceedings{barkataki2021artificial,
title = {Artificial Neural Network based automatic classification of target shapes from GPR data},
author = {Nairit Barkataki and Anirban Bhattacharjee and Sharmistha Mazumdar and Banty Tiru and Utpal Sarma},
url = {https://nairit.in/wp-content/uploads/2022/12/152_ANNTargetShapeGPR_CR.pdf},
doi = {10.1109/CSITSS54238.2021.9682980},
year = {2021},
date = {2021-12-01},
urldate = {2021-12-01},
booktitle = {2021 IEEE International Conference on Computation System and Information Technology for Sustainable Solutions (CSITSS)},
organization = {IEEE},
keywords = {Artificial Neural Network, classification, Deep learning, Ground penetrating radar, object shape},
pubstate = {published},
tppubtype = {inproceedings}
}
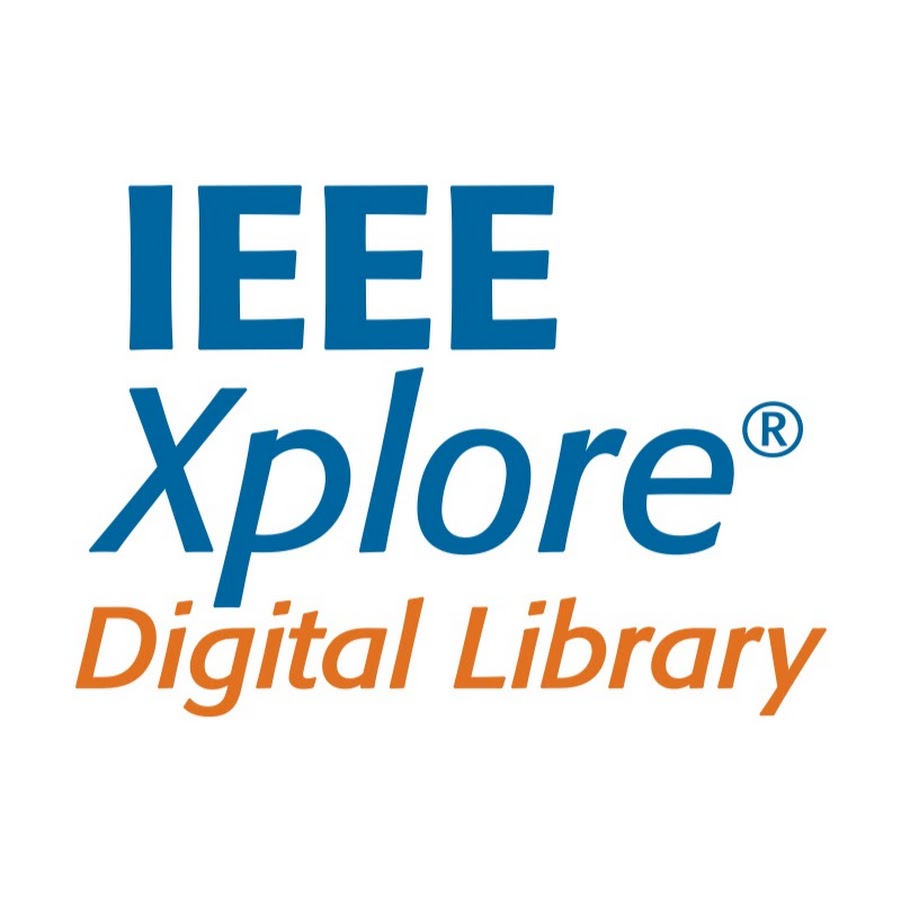
Nairit Barkataki; Sharmistha Mazumdar; P Bipasha Devi Singha; Jyoti Kumari; Banty Tiru; Utpal Sarma
Classification of soil types from GPR B Scans using deep learning techniques Proceedings Article
In: 2021 6th IEEE International Conference on Recent Trends in Electronics, Information & Communication Technology (RTEICT 2021), pp. 840–844, IEEE 2021.
BibTeX | Tags: classification, Deep learning, Ground penetrating radar, Soil Type | Links:
@inproceedings{barkataki2021classificationsoil,
title = {Classification of soil types from GPR B Scans using deep learning techniques},
author = {Nairit Barkataki and Sharmistha Mazumdar and P Bipasha Devi Singha and Jyoti Kumari and Banty Tiru and Utpal Sarma},
url = {https://nairit.in/wp-content/uploads/2022/12/Paper004_IRTEICT_SoilType.pdf},
doi = {10.1109/RTEICT52294.2021.9573702},
year = {2021},
date = {2021-08-01},
urldate = {2021-08-01},
booktitle = {2021 6th IEEE International Conference on Recent Trends in Electronics, Information & Communication Technology (RTEICT 2021)},
pages = {840--844},
organization = {IEEE},
keywords = {classification, Deep learning, Ground penetrating radar, Soil Type},
pubstate = {published},
tppubtype = {inproceedings}
}
2020
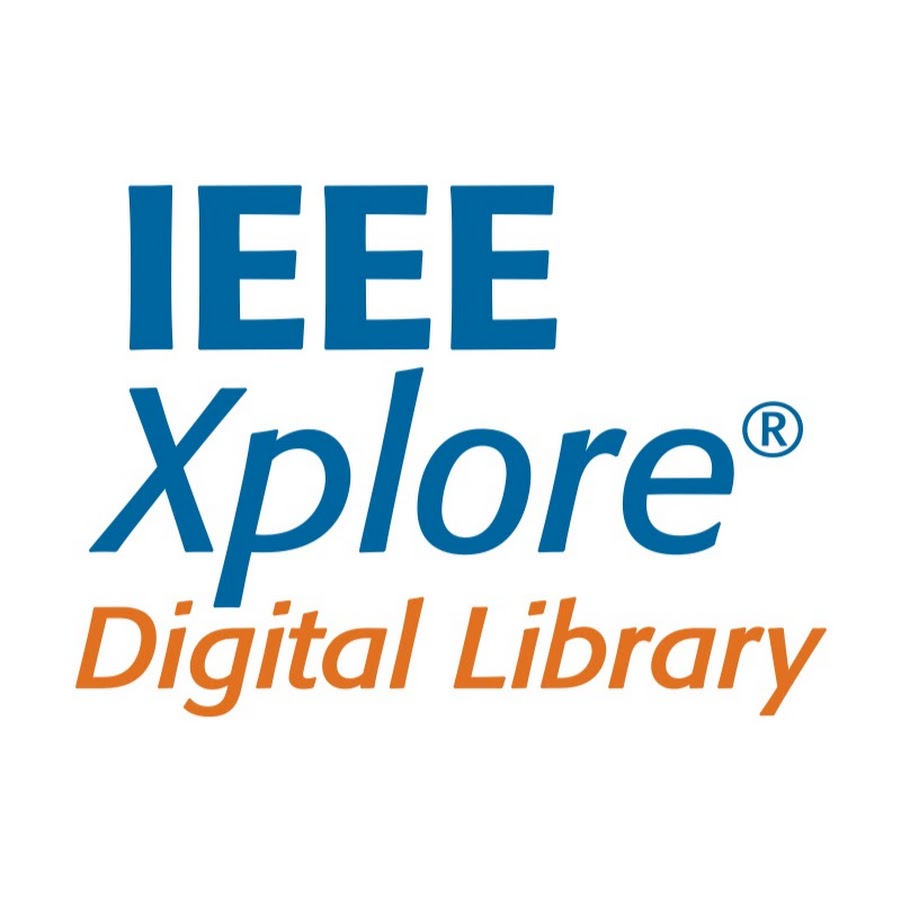
Nairit Barkataki; Sharmistha Mazumdar; Rajdeep Talukdar; Priyanka Chakraborty; Banty Tiru; Utpal Sarma
Prediction of Size of Buried Objects using Ground Penetrating Radar and Machine Learning Techniques Proceedings Article
In: 2020 International Conference on Computational Performance Evaluation (ComPE), pp. 781-785, IEEE 2020.
Abstract | BibTeX | Tags: classification, Ground penetrating radar, machine learning, object size prediction | Links:
@inproceedings{barkataki2020Prediction,
title = {Prediction of Size of Buried Objects using Ground Penetrating Radar and Machine Learning Techniques},
author = {Nairit Barkataki and Sharmistha Mazumdar and Rajdeep Talukdar and Priyanka Chakraborty and Banty Tiru and Utpal Sarma},
url = {https://nairit.in/wp-content/uploads/2022/12/PID6437207_Prediction-of-Nairit.pdf},
doi = {10.1109/ComPE49325.2020.9200094},
year = {2020},
date = {2020-07-01},
urldate = {2020-07-01},
booktitle = {2020 International Conference on Computational Performance Evaluation (ComPE)},
pages = {781-785},
organization = {IEEE},
abstract = {Ground penetrating radar (GPR) uses electromagnetic (EM) wave to detect the subsurface objects. Interpretation and analysis of GPR signals are still challenging tasks as it requires skilled user (geologists in most cases). Particularly difficult is the prediction of the object sizes. This paper proposes a new method for predicting size of buried objects. First, standard scaling pre-processing techniques are used to optimise the B-Scan data. The features are then supplied to Random Forest (RF) and Support Vector Machine (SVM) classifiers to automatically predict the size of the buried object. The proposed feature based RF classifier shows similar performance in the accuracy of classification compared to SVM (Radial Basis Function kernel) system.},
keywords = {classification, Ground penetrating radar, machine learning, object size prediction},
pubstate = {published},
tppubtype = {inproceedings}
}