Publications
Journal Articles
Sorry, no publications matched your criteria.
Conference Proceedings
2020
1.
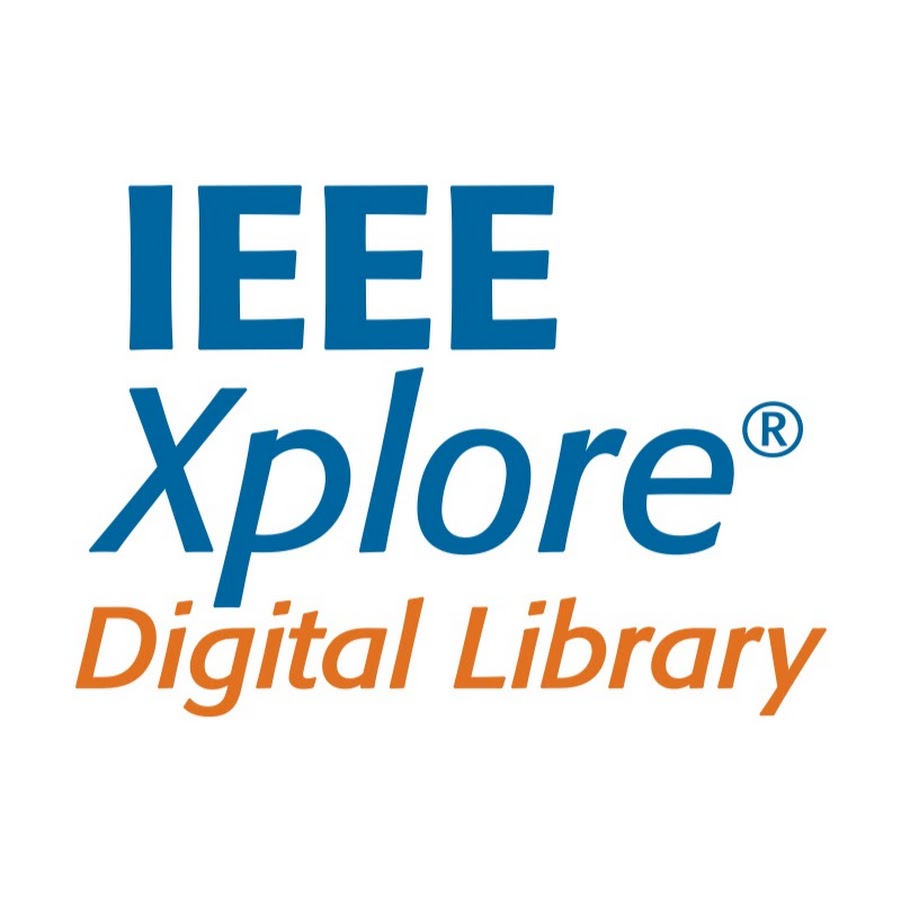
Nairit Barkataki; Sharmistha Mazumdar; Rajdeep Talukdar; Priyanka Chakraborty; Banty Tiru; Utpal Sarma
Prediction of Size of Buried Objects using Ground Penetrating Radar and Machine Learning Techniques Proceedings Article
In: 2020 International Conference on Computational Performance Evaluation (ComPE), pp. 781-785, IEEE 2020.
Abstract | BibTeX | Tags: classification, Ground penetrating radar, machine learning, object size prediction | Links:
@inproceedings{barkataki2020Prediction,
title = {Prediction of Size of Buried Objects using Ground Penetrating Radar and Machine Learning Techniques},
author = {Nairit Barkataki and Sharmistha Mazumdar and Rajdeep Talukdar and Priyanka Chakraborty and Banty Tiru and Utpal Sarma},
url = {https://nairit.in/wp-content/uploads/2022/12/PID6437207_Prediction-of-Nairit.pdf},
doi = {10.1109/ComPE49325.2020.9200094},
year = {2020},
date = {2020-07-01},
urldate = {2020-07-01},
booktitle = {2020 International Conference on Computational Performance Evaluation (ComPE)},
pages = {781-785},
organization = {IEEE},
abstract = {Ground penetrating radar (GPR) uses electromagnetic (EM) wave to detect the subsurface objects. Interpretation and analysis of GPR signals are still challenging tasks as it requires skilled user (geologists in most cases). Particularly difficult is the prediction of the object sizes. This paper proposes a new method for predicting size of buried objects. First, standard scaling pre-processing techniques are used to optimise the B-Scan data. The features are then supplied to Random Forest (RF) and Support Vector Machine (SVM) classifiers to automatically predict the size of the buried object. The proposed feature based RF classifier shows similar performance in the accuracy of classification compared to SVM (Radial Basis Function kernel) system.},
keywords = {classification, Ground penetrating radar, machine learning, object size prediction},
pubstate = {published},
tppubtype = {inproceedings}
}
Ground penetrating radar (GPR) uses electromagnetic (EM) wave to detect the subsurface objects. Interpretation and analysis of GPR signals are still challenging tasks as it requires skilled user (geologists in most cases). Particularly difficult is the prediction of the object sizes. This paper proposes a new method for predicting size of buried objects. First, standard scaling pre-processing techniques are used to optimise the B-Scan data. The features are then supplied to Random Forest (RF) and Support Vector Machine (SVM) classifiers to automatically predict the size of the buried object. The proposed feature based RF classifier shows similar performance in the accuracy of classification compared to SVM (Radial Basis Function kernel) system.