Publications
Journal Articles
2023

Nairit Barkataki; Banty Tiru; Utpal Sarma
Size estimation of underground targets from GPR frequency spectra: A deep learning approach Journal Article
In: Journal of Applied Geophysics, vol. 213, pp. 105009, 2023, ISSN: 0926-9851.
Abstract | BibTeX | Tags: Archeology, Artificial Neural Network, Bridge inspection, Civil Engineering, Deep learning, Ground penetrating radar, neural networks, object size prediction | Links:
@article{barkataki2023size,
title = {Size estimation of underground targets from GPR frequency spectra: A deep learning approach},
author = {Nairit Barkataki and Banty Tiru and Utpal Sarma},
url = {https://nairit.in/wp-content/uploads/Paper009_JAG_ObjectSizeAScan.pdf},
doi = {10.1016/j.jappgeo.2023.105009},
issn = {0926-9851},
year = {2023},
date = {2023-06-01},
urldate = {2023-06-01},
journal = {Journal of Applied Geophysics},
volume = {213},
pages = {105009},
abstract = {GPR (Ground Penetrating Radar) is a robust and effective device for identifying underground artefacts. Construction companies and civil engineers should be aware of the sizes of rebars and pipelines before and during construction work for various reasons. Most research efforts have typically concentrated on GPR signal analysis in the time domain, however recent studies have increasingly focused on analysis in the frequency domain. This paper proposes an artificial neural network (ANN) model for estimating the diameter of underground rods (solid) and pipes (hollow). GPR data captured in the time domain domain is transformed to the frequency domain using FFT, after which feature extraction is performed using ANN. An FPGA-based prototype GPR system is used to collect GPR A-Scan data for a variety of targets made of aluminium, stainless steel, rebar, and PVC. A mean absolute percentage error of 1.89% is achieved using the proposed model. The experimental results confirm the effectiveness of the proposed approach in extracting size-related information from GPR data.},
keywords = {Archeology, Artificial Neural Network, Bridge inspection, Civil Engineering, Deep learning, Ground penetrating radar, neural networks, object size prediction},
pubstate = {published},
tppubtype = {article}
}
2022

Nairit Barkataki; Banty Tiru; Utpal Sarma
A CNN model for predicting size of buried objects from GPR B-Scans Journal Article
In: Journal of Applied Geophysics, vol. 200, pp. 104620, 2022, ISSN: 0926-9851.
BibTeX | Tags: CNN, Deep learning, Ground penetrating radar, object size prediction | Links:
@article{barkataki2022cnn,
title = {A CNN model for predicting size of buried objects from GPR B-Scans},
author = {Nairit Barkataki and Banty Tiru and Utpal Sarma},
url = {https://nairit.in/wp-content/uploads/2023/01/Paper003_JAG_ObjectSizeDL.pdf},
doi = {10.1016/j.jappgeo.2022.104620},
issn = {0926-9851},
year = {2022},
date = {2022-01-01},
urldate = {2022-01-01},
journal = {Journal of Applied Geophysics},
volume = {200},
pages = {104620},
publisher = {Elsevier},
keywords = {CNN, Deep learning, Ground penetrating radar, object size prediction},
pubstate = {published},
tppubtype = {article}
}
Conference Proceedings
2022
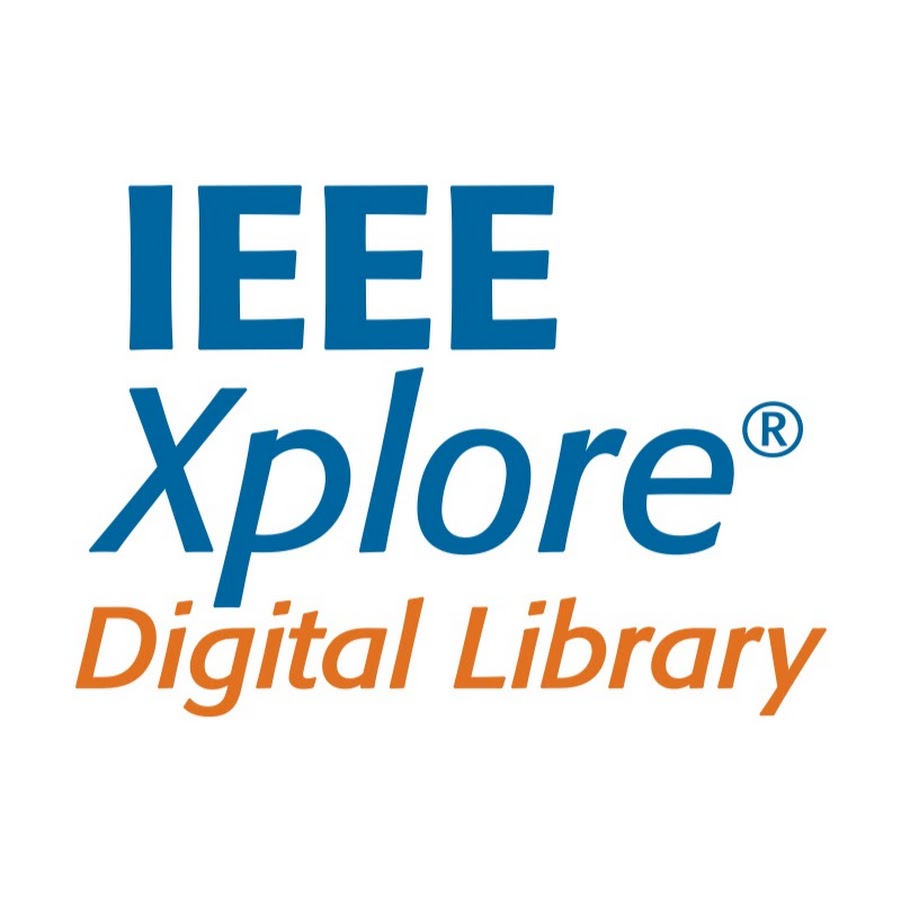
Nairit Barkataki; Ankur Jyoti Kalita; Utpal Sarma
Automatic Material Classification of Targets from GPR Data using Artificial Neural Networks Proceedings Article
In: 2022 IEEE Silchar Subsection Conference (SILCON), IEEE 2022, ISBN: 978-1-6654-7100-8.
Abstract | BibTeX | Tags: Archeology, Artificial Neural Network, classification, Deep learning, Ground penetrating radar, Landmine detection, Object Material | Links:
@inproceedings{barkataki2022automatic,
title = {Automatic Material Classification of Targets from GPR Data using Artificial Neural Networks},
author = {Nairit Barkataki and Ankur Jyoti Kalita and Utpal Sarma},
url = {https://nairit.in/wp-content/uploads/2022_SILCON_Barkataki_Material.pdf},
doi = {10.1109/SILCON55242.2022.10028944},
isbn = {978-1-6654-7100-8},
year = {2022},
date = {2022-11-06},
urldate = {2022-11-06},
booktitle = {2022 IEEE Silchar Subsection Conference (SILCON)},
journal = {2022 IEEE Silchar Subsection Conference (SILCON)},
organization = {IEEE},
abstract = {Ground penetrating radar (GPR) is a preferred non-destructive method to study and identify buried objects in the field of geology, civil engineering, archaeology, military, etc. Landmines are now largely composed of plastic and other non-metallic materials, while archaeologists must deal with buried artefacts such as ceramics, pillars, and walls built of a range of materials. As a result, understanding the material properties of buried artefacts is critical. This study presents an ANN model for automatic classification of buried objects from GPR A-Scan data. The proposed ANN model is trained and validated using a synthetic dataset generated using gprMax. The model performs well in classifying three different object classes of aluminium, iron and limestone, while achieving an overall accuracy of 95%.},
keywords = {Archeology, Artificial Neural Network, classification, Deep learning, Ground penetrating radar, Landmine detection, Object Material},
pubstate = {published},
tppubtype = {inproceedings}
}
2021
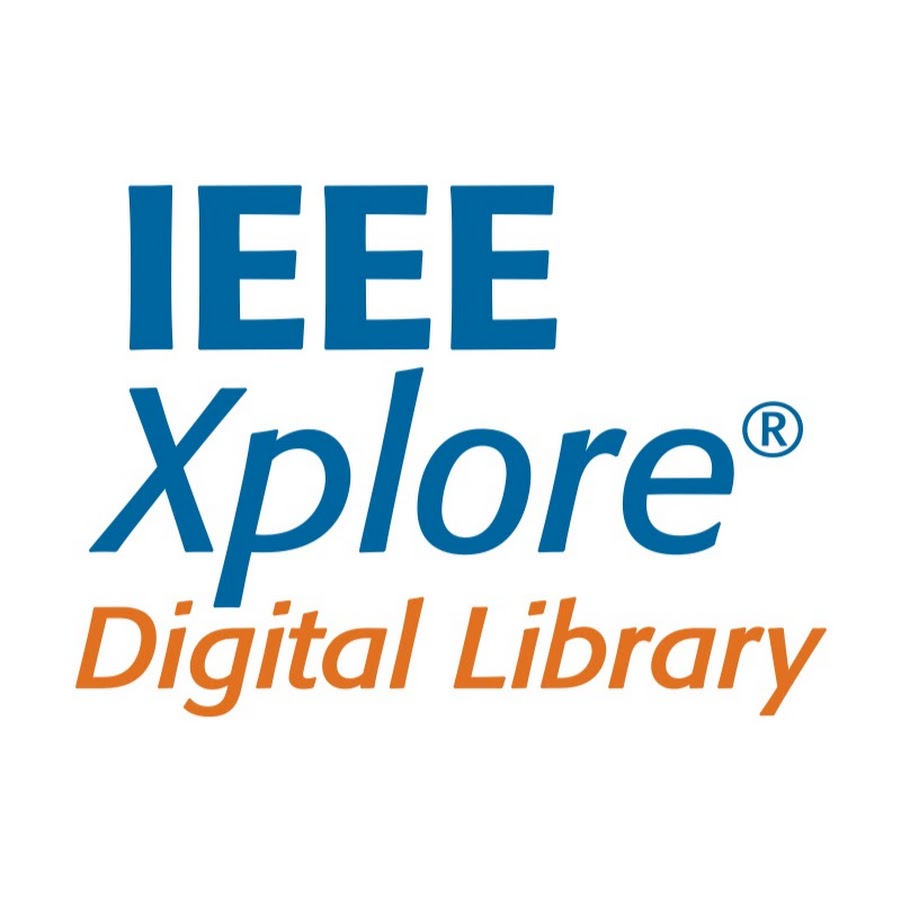
Nairit Barkataki; Sharmistha Mazumdar; Banty Tiru; Utpal Sarma
Estimation of soil moisture from GPR data using artificial neural networks Proceedings Article
In: 2021 IEEE International Conference on Technology, Research, and Innovation for Betterment of Society (TRIBES), IEEE 2021, ISBN: 978-1-6654-3342-6.
Abstract | BibTeX | Tags: Artificial Neural Network, Deep learning, Ground penetrating radar, Soil Moisture | Links:
@inproceedings{barkataki2021estimationsoil,
title = {Estimation of soil moisture from GPR data using artificial neural networks},
author = {Nairit Barkataki and Sharmistha Mazumdar and Banty Tiru and Utpal Sarma},
url = {https://nairit.in/wp-content/uploads/2022/12/149_Barkataki.pdf},
doi = {10.1109/TRIBES52498.2021.9751623},
isbn = {978-1-6654-3342-6},
year = {2021},
date = {2021-12-19},
urldate = {2021-12-19},
booktitle = {2021 IEEE International Conference on Technology, Research, and Innovation for Betterment of Society (TRIBES)},
organization = {IEEE},
abstract = {Soil moisture estimation is essential for understanding the water cycle and its impact on weather and climate. GPR based soil moisture estimation is non-invasive in nature and provides quicker results as compared to standard laboratory approaches. Deep learning algorithms have been shown to be an effective method for extracting characteristics from GPR data. To assess soil moisture, this paper offers a deep artificial neural network (ANN) model. The data set was created using finite-difference-time-domain (FDTD) simulation and is used to train and validate the ANN model. The suggested model predicts soil moisture from GPR A-Scans well, with R2 = 0.998 and mean average percentage error (MAPE) of 1.23.},
keywords = {Artificial Neural Network, Deep learning, Ground penetrating radar, Soil Moisture},
pubstate = {published},
tppubtype = {inproceedings}
}
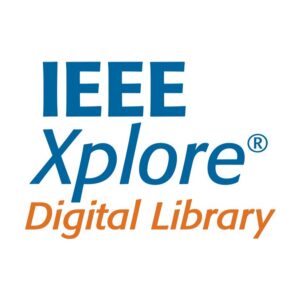
Nairit Barkataki; Anirban Bhattacharjee; Sharmistha Mazumdar; Banty Tiru; Utpal Sarma
Artificial Neural Network based automatic classification of target shapes from GPR data Best Paper Proceedings Article
In: 2021 IEEE International Conference on Computation System and Information Technology for Sustainable Solutions (CSITSS), IEEE 2021.
BibTeX | Tags: Artificial Neural Network, classification, Deep learning, Ground penetrating radar, object shape | Links:
@inproceedings{barkataki2021artificial,
title = {Artificial Neural Network based automatic classification of target shapes from GPR data},
author = {Nairit Barkataki and Anirban Bhattacharjee and Sharmistha Mazumdar and Banty Tiru and Utpal Sarma},
url = {https://nairit.in/wp-content/uploads/2022/12/152_ANNTargetShapeGPR_CR.pdf},
doi = {10.1109/CSITSS54238.2021.9682980},
year = {2021},
date = {2021-12-01},
urldate = {2021-12-01},
booktitle = {2021 IEEE International Conference on Computation System and Information Technology for Sustainable Solutions (CSITSS)},
organization = {IEEE},
keywords = {Artificial Neural Network, classification, Deep learning, Ground penetrating radar, object shape},
pubstate = {published},
tppubtype = {inproceedings}
}
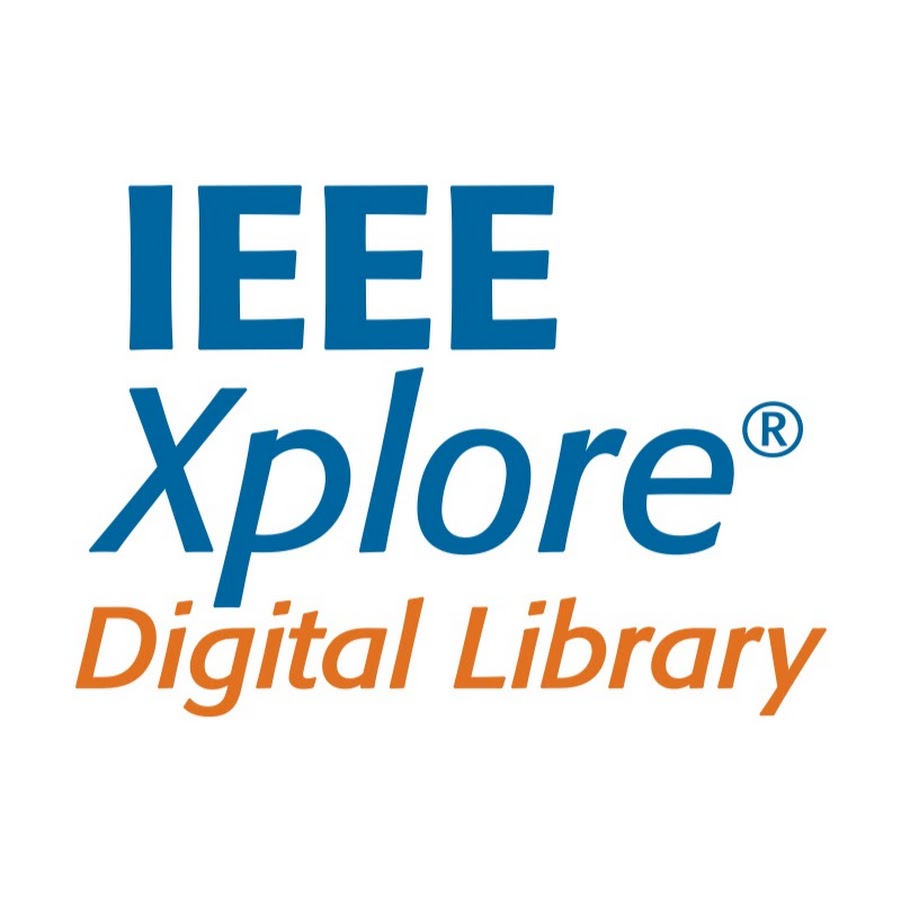
Nairit Barkataki; Sharmistha Mazumdar; P Bipasha Devi Singha; Jyoti Kumari; Banty Tiru; Utpal Sarma
Classification of soil types from GPR B Scans using deep learning techniques Proceedings Article
In: 2021 6th IEEE International Conference on Recent Trends in Electronics, Information & Communication Technology (RTEICT 2021), pp. 840–844, IEEE 2021.
BibTeX | Tags: classification, Deep learning, Ground penetrating radar, Soil Type | Links:
@inproceedings{barkataki2021classificationsoil,
title = {Classification of soil types from GPR B Scans using deep learning techniques},
author = {Nairit Barkataki and Sharmistha Mazumdar and P Bipasha Devi Singha and Jyoti Kumari and Banty Tiru and Utpal Sarma},
url = {https://nairit.in/wp-content/uploads/2022/12/Paper004_IRTEICT_SoilType.pdf},
doi = {10.1109/RTEICT52294.2021.9573702},
year = {2021},
date = {2021-08-01},
urldate = {2021-08-01},
booktitle = {2021 6th IEEE International Conference on Recent Trends in Electronics, Information & Communication Technology (RTEICT 2021)},
pages = {840--844},
organization = {IEEE},
keywords = {classification, Deep learning, Ground penetrating radar, Soil Type},
pubstate = {published},
tppubtype = {inproceedings}
}