Publications
Journal Articles
Sorry, no publications matched your criteria.
Conference Proceedings
2021
1.
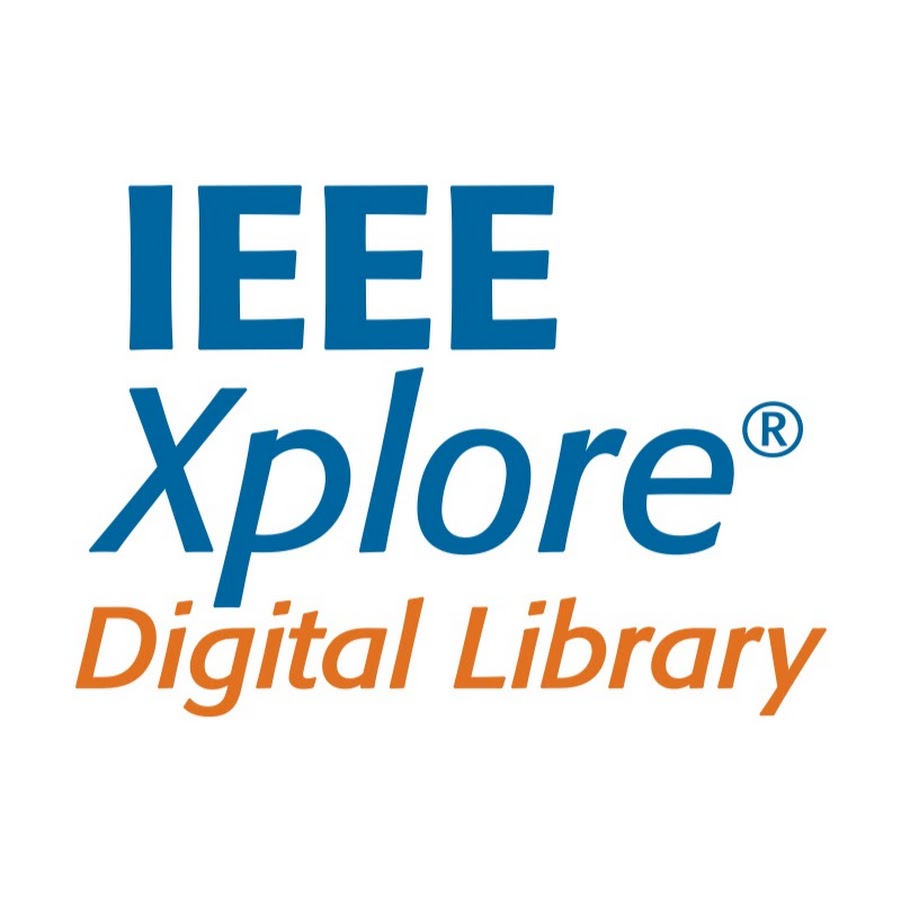
Nairit Barkataki; Sharmistha Mazumdar; Banty Tiru; Utpal Sarma
Estimation of soil moisture from GPR data using artificial neural networks Proceedings Article
In: 2021 IEEE International Conference on Technology, Research, and Innovation for Betterment of Society (TRIBES), IEEE 2021, ISBN: 978-1-6654-3342-6.
Abstract | BibTeX | Tags: Artificial Neural Network, Deep learning, Ground penetrating radar, Soil Moisture | Links:
@inproceedings{barkataki2021estimationsoil,
title = {Estimation of soil moisture from GPR data using artificial neural networks},
author = {Nairit Barkataki and Sharmistha Mazumdar and Banty Tiru and Utpal Sarma},
url = {https://nairit.in/wp-content/uploads/2022/12/149_Barkataki.pdf},
doi = {10.1109/TRIBES52498.2021.9751623},
isbn = {978-1-6654-3342-6},
year = {2021},
date = {2021-12-19},
urldate = {2021-12-19},
booktitle = {2021 IEEE International Conference on Technology, Research, and Innovation for Betterment of Society (TRIBES)},
organization = {IEEE},
abstract = {Soil moisture estimation is essential for understanding the water cycle and its impact on weather and climate. GPR based soil moisture estimation is non-invasive in nature and provides quicker results as compared to standard laboratory approaches. Deep learning algorithms have been shown to be an effective method for extracting characteristics from GPR data. To assess soil moisture, this paper offers a deep artificial neural network (ANN) model. The data set was created using finite-difference-time-domain (FDTD) simulation and is used to train and validate the ANN model. The suggested model predicts soil moisture from GPR A-Scans well, with R2 = 0.998 and mean average percentage error (MAPE) of 1.23.},
keywords = {Artificial Neural Network, Deep learning, Ground penetrating radar, Soil Moisture},
pubstate = {published},
tppubtype = {inproceedings}
}
Soil moisture estimation is essential for understanding the water cycle and its impact on weather and climate. GPR based soil moisture estimation is non-invasive in nature and provides quicker results as compared to standard laboratory approaches. Deep learning algorithms have been shown to be an effective method for extracting characteristics from GPR data. To assess soil moisture, this paper offers a deep artificial neural network (ANN) model. The data set was created using finite-difference-time-domain (FDTD) simulation and is used to train and validate the ANN model. The suggested model predicts soil moisture from GPR A-Scans well, with R2 = 0.998 and mean average percentage error (MAPE) of 1.23.