Publications
Journal Articles
Sorry, no publications matched your criteria.
Conference Proceedings
2022
1.
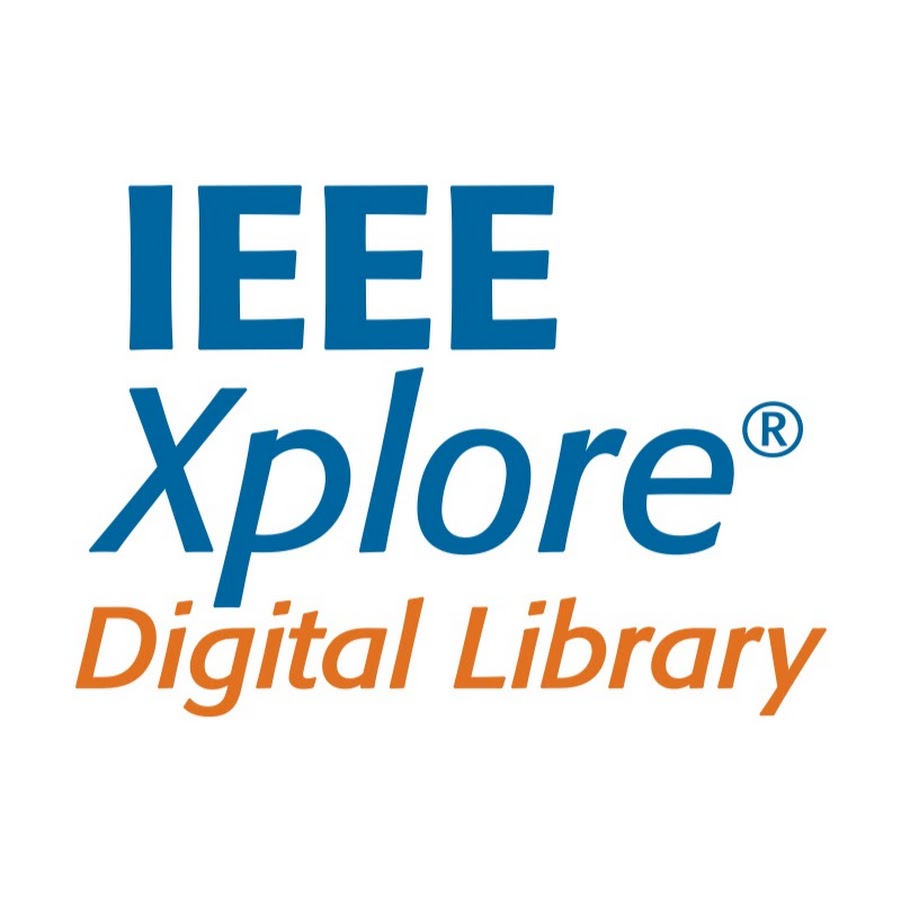
Nairit Barkataki; Ankur Jyoti Kalita; Utpal Sarma
Automatic Material Classification of Targets from GPR Data using Artificial Neural Networks Proceedings Article
In: 2022 IEEE Silchar Subsection Conference (SILCON), IEEE 2022, ISBN: 978-1-6654-7100-8.
Abstract | BibTeX | Tags: Archeology, Artificial Neural Network, classification, Deep learning, Ground penetrating radar, Landmine detection, Object Material | Links:
@inproceedings{barkataki2022automatic,
title = {Automatic Material Classification of Targets from GPR Data using Artificial Neural Networks},
author = {Nairit Barkataki and Ankur Jyoti Kalita and Utpal Sarma},
url = {https://nairit.in/wp-content/uploads/2022_SILCON_Barkataki_Material.pdf},
doi = {10.1109/SILCON55242.2022.10028944},
isbn = {978-1-6654-7100-8},
year = {2022},
date = {2022-11-06},
urldate = {2022-11-06},
booktitle = {2022 IEEE Silchar Subsection Conference (SILCON)},
journal = {2022 IEEE Silchar Subsection Conference (SILCON)},
organization = {IEEE},
abstract = {Ground penetrating radar (GPR) is a preferred non-destructive method to study and identify buried objects in the field of geology, civil engineering, archaeology, military, etc. Landmines are now largely composed of plastic and other non-metallic materials, while archaeologists must deal with buried artefacts such as ceramics, pillars, and walls built of a range of materials. As a result, understanding the material properties of buried artefacts is critical. This study presents an ANN model for automatic classification of buried objects from GPR A-Scan data. The proposed ANN model is trained and validated using a synthetic dataset generated using gprMax. The model performs well in classifying three different object classes of aluminium, iron and limestone, while achieving an overall accuracy of 95%.},
keywords = {Archeology, Artificial Neural Network, classification, Deep learning, Ground penetrating radar, Landmine detection, Object Material},
pubstate = {published},
tppubtype = {inproceedings}
}
Ground penetrating radar (GPR) is a preferred non-destructive method to study and identify buried objects in the field of geology, civil engineering, archaeology, military, etc. Landmines are now largely composed of plastic and other non-metallic materials, while archaeologists must deal with buried artefacts such as ceramics, pillars, and walls built of a range of materials. As a result, understanding the material properties of buried artefacts is critical. This study presents an ANN model for automatic classification of buried objects from GPR A-Scan data. The proposed ANN model is trained and validated using a synthetic dataset generated using gprMax. The model performs well in classifying three different object classes of aluminium, iron and limestone, while achieving an overall accuracy of 95%.